A new computational method for reconstructing the evolution of a tumor
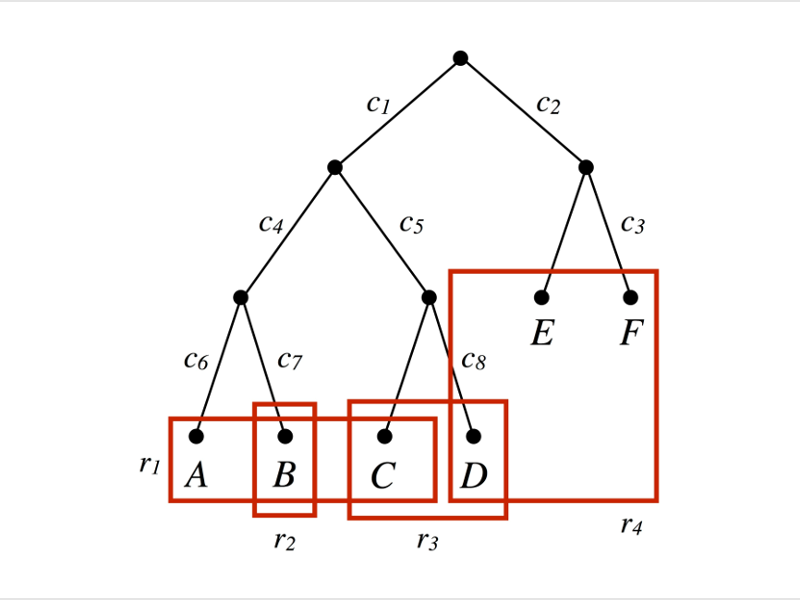
Discovering the evolution of a tumor may help identify driver mutations and provide a more comprehensive view on the history of the tumor. One way to tackle this problem is to take several samples from the tumor, find the mutations they contain, and then determine a plausible evolution of the tumor that gave rise to these mutations.
A multi-disciplinary team lead by University Researcher Alexandru Tomescu from the University of Helsinki and Prof. Martin Milanič from the University of Primorska (Slovenia) has developed a new method for discovering the evolution of the tumor, using DNA sequencing data from multiple samples of a tumor. This method proved more accurate than the existing methods, both on synthetic data and on data from several types for cancer, such as clear cell renal cell carcinoma, high-grade serous ovarian cancer, breast cancer xenoengraftment and uterine leiomyomas.
This research has been published in the journal Bioinformatics, and is available under an Open Access license: Edin Husić, Xinyue Li, Ademir Hujdurović, Miika Mehine, Romeo Rizzi, Veli Mäkinen, Martin Milanič and Alexandru I Tomescu, MIPUP: Minimum perfect unmixed phylogenies for multi-sampled tumors via branchings and ILP.
This computational method is based on a previous theoretical result proving a relation between this bioinformatics problem and a problem on directed graphs, presented at the WG 2017 graph-theory conference (Ademir Hujdurović, Edin Husić, Martin Milanič, Romeo Rizzi, Alexandru I. Tomescu, The Minimum Conflict-Free Row Split Problem Revisited. WG 2017: 303-315) and published in the journal ACM Transactions on Algorithms (Ademir Hujdurovic, Edin Husic, Martin Milanic, Romeo Rizzi, Alexandru I. Tomescu, Perfect Phylogenies via Branchings in Acyclic Digraphs and a Generalization of Dilworth’s Theorem. ACM Trans. Algorithms 14(2): 20:1-20:26 (2018)).
An example is shown in the figure above: Tumors usually have a tree-like evolution, with new mutations (c1, c2, c3, …) accumulating on each edge of the tree. DNA sequencing samples (r1, r2, r3, r4) usually mix several leaves (A, B, C, D, E) of the tree, making it hard to discover the actual evolution.
The project was partly supported by the HIIT FCHealth Programme.