MIT Professor Tommi Jaakkola shared his wisdom on applying AI in other fields
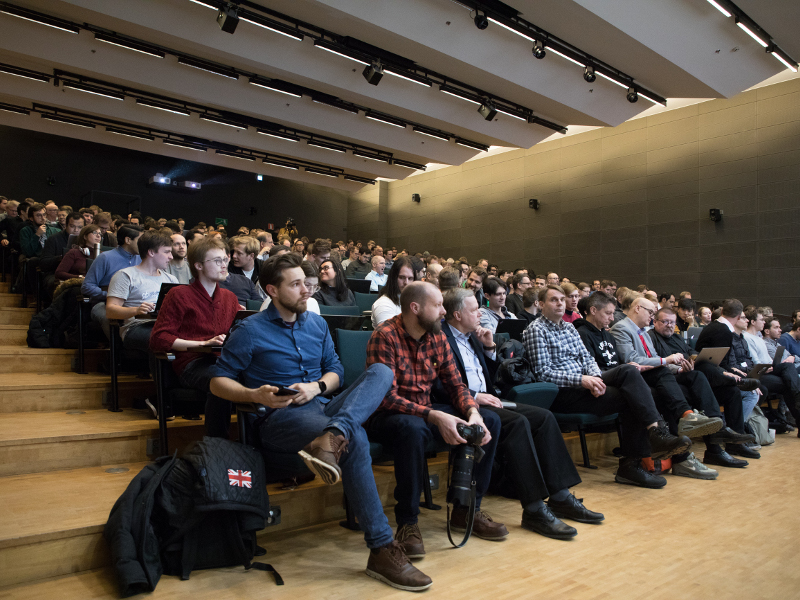
The Helsinki Distinguished Lecture Series on Future Information Technology got an excellent start for 2019, when the large auditorium of the Otaniemi TUAS building was packed full for Tommi Jaakkola‘s talk. He is a world class researcher and an acclaimed teacher whose work focuses on both foundational theory and applications of machine learning. He received his Master’s degree from Helsinki University of Technology, did his PhD at MIT, and joined the MIT faculty in 1998.
The talk, titled “Modeling with Machine Learning: Challenges and Some Solutions”, consisted of two parts. The first part illustrated how AI can be used as a tool to accelerate and transform other areas of science and engineering. By enabling complex inferences to be made from data, machine learning extends the reach of modeling to phenomena that are not well-understood yet. The second part of the talk gave an overview of efforts to make machine learning models more interpretable. While major advances have been made in achieving good performance in complex tasks, understanding how the models work is often difficult even for an expert. These two challenges, construction of sophisticated models and improving interpretability, are typically seen as two different subfields of machine learning research, but one of the main conclusions of the talk was that significant synergy is emerging.
To demonstrate how AI can accelerate progress in other areas, Professor Jaakkola presented some of the work he and his collaborators have done in chemistry. Vast amounts of underused information exist in databases, literature, and researchers’ notebooks. In an attempt to accelerate drug design, they have created models that predict the properties of a molecule on the basis its structure. They have also worked on predicting the major products of chemical reactions, achieving a level of performance on par with human experts.
Their approach to improving interpretability is based on the observation that although a full understanding of a complex model cannot be simple, there are ways to facilitate “local” understanding of how individual inputs are processed. This can be done even with existing models by repeatedly modifying the input and observing the effect on the output. When creating new models, constraints can be applied to internal structures to make them locally interpretable.
One of the key challenges in machine learning is to get beyond the training data with models that capture fundamental aspects of the domain. In drug design, for example, computational exploration of new chemical spaces would be even more valuable than working within the boundaries of the chemical diversity present in the data. Jaakkola proposes incorporating more domain knowledge, such as integrated physics calculations, in machine learning methods to achieve this kind of deep generalizations.
The importance of domain knowledge has implications on how research and education in AI should be organized. Jaakkola stated that taking AI successfully into other fields can only be done by “teams of three”: an AI expert, a domain expert, and a person knowledgeable about both. Here in Finland, the research agenda of FCAI is based on similar ideas of cross-disciplinary collaboration.
Applying AI Across Fields also means that a broader variety of people should have access to relevant education. At MIT, Professor Jaakkola is teaching a course titled Introduction to Machine Learning, which has become popular among students in other disciplines besides computer science. Alexander Jung has had a lot of success with a similar course at Aalto University, and Elements of AI, led by Teemu Roos, targets an even broader audience with the objective of educating 1% of the Finnish population in the basics of AI.
A video recording of Tommi Jaakola’s talk is available and highly recommended to everyone.