New machine learning method enables deeper understanding of pathogenic bacteria
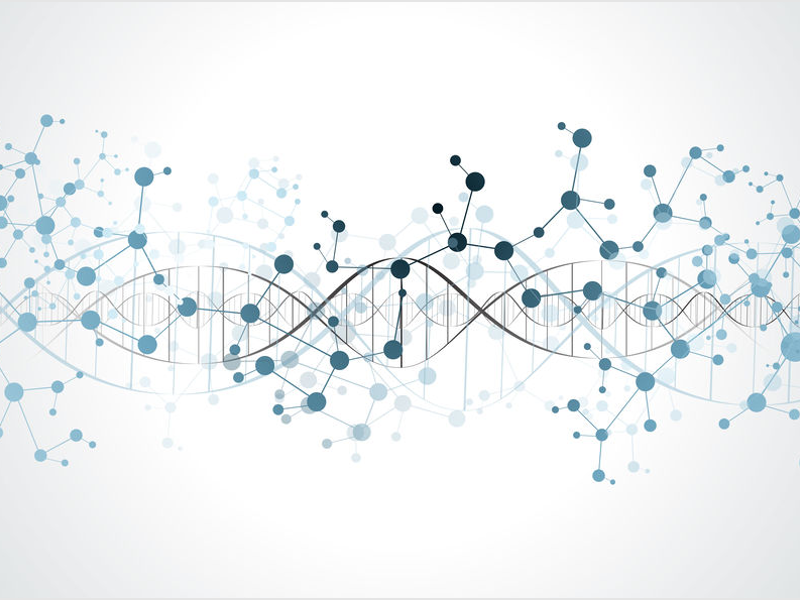
A research team led by professor Jukka Corander has created a new machine learning method for the analysis of bacterial genomes. Thanks to its computational efficiency, the new method can handle an order of magnitude larger data sets than the previous methods, and is applicable at the level of pangenomes of most pathogenic bacteria. The current focus of the team is on the pneumococcus and the meningococcus, which are major causes of life-threatening diseases such as pneumonia, sepsis and meningitis, and frequently cause also other milder diseases. Their results display important findings on the evolution of both virulence and antibiotic resistance in populations of these bacteria. The research is part of professor Corander’s ERC AdG project which develops scalable inference methods for infectious disease epidemiology.
A manuscript describing the method and the first results is available at bioRxiv.