HIIT Postdoctoral Fellows 2020
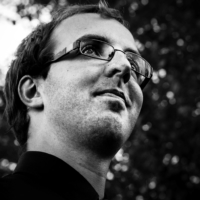
Jeremias Berg
https://jeremiasberg.com/
Dr. Jeremias Berg obtained his Master’s degree in Mathematics in 2014 and his PhD in Computer Science in 2018 from the University of Helsinki. His doctoral studies were supervised by Professor Matti Järvisalo. Berg’s doctoral research and thesis “Solving Optimization Problems via Maximum Satisfiability: Encodings and Re-Encodings“ received both national and international recognition in the form of awards from both the University of Helsinki and the Association for Constraint Programming. After graduating Dr. Berg undertook two research visits: to the University of Melbourne in 2018 and to the University of Toronto in 2019. Both visits were fruitful, resulting in publications and ongoing research collaboration. Currently, Berg is working as an HIIT Postdoctoral Fellow in the Constraint Reasoning and Optimisation group at the University of Helsinki. Berg is actively teaching at the university, for example, supervising Bachelor’s and Master’s theses. Berg also contributes to the University’s core duty of community relations, for example via his membership in the Young Academy Finland (Nuorten Tiedeakatemia). More information regarding Berg and his research can be found on his webpage https://jeremiasberg.com/.
Berg’s research focuses on developing effective, general-purpose, tools for solving NP-hard optimization problems arising in data analysis, machine learning and AI. He is especially interested in logic-based declarative approaches. His doctoral research focused on the Boolean optimization paradigm of Maximum Satisfiability (MaxSAT). The research developed the theoretical and algorithmic understanding of MaxSAT. It also resulted in state-of-the-art MaxSAT-based solution methods to central data-analysis problems, including data-visualisation, clustering and Bayesian network structure learning. Berg’s more recent research builds on his doctoral research. His recent interests include incomplete solving, i.e. developing algorithms for quickly computing good solutions to difficult optimisation problems. He is the primary developer of the state-of-the-art incomplete MaxSAT solver Loandra – one of the best performing solvers in the recent MaxSAT Evaluations. His research has also contributed to numerous other tools for solving NP-hard problems: including the CP solver solver Geas, the MaxSAT preprocessor MaxPre, and Triangulator, an implementation of the Bouchitté-Todinca Algorithm for solving various graph optimisation problems.
Berg’s recent research highlights include the 2020 Christian Schulte Doctoral Award, awarded each year to one doctoral thesis world-wide by Association for Constraint Programming, the primary international representative association in constraint programming. Another highlight from the recent year is the best paper award of the 2020 SAT conference for his paper Abstract Cores in Implicit Hitting Set MaxSat Solving co-authored with Fahiem Bachhus and Alex Poole. The paper summarised work begun during his visit to Toronto in 2019. Finally, Berg’s MaxSAT solver Loandra performed very well in the 2020 MaxSAT Evaluations that Berg also helped to organise. In 2020, he was also invited to be a member of Young Academy of Finland and participated in its “meet a researcher” initiative.
Sample of recent publications:
Berg, J., Bacchus, F. & Poole, A., Abstract cores in implicit hitting set MaxSat solving, 2020, Proc. 23rd International Conference on Theory and Applications of Satisfiability Testing (SAT 2020), p. 277-294.
Grange, G., Berg, J., Demirovic, E. & Stuckey, P., Core-guided and core-boosted search for CP, Proc. 17th International Conference on Integration of Constraint Programming, Artificial Intelligence, and Operations Research, p. 205-221.
Leivo, M. J., Berg, J. & Järvisalo, M., Preprocssing in incomplete MaxSAT solving, Proc. 24th European Conference on Artificial Intelligence (ECAI 2020), p. 347-354.
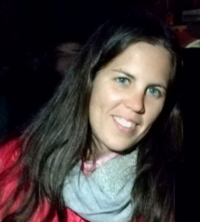
Laia Amoros Carafi
Dr. Carafi is part of the Machine Learning for Big Data Research Group of Alex Jung and she work in close connection with the Algebra, Number Theory and Applications Research Group of Camilla Hollanti.
Carafi has a BSc and a MSc in mathematics from the University of Barcelona. In 2016 she obtained a PhD in number theory under a collaboration between the University of Barcelona and the University of Luxembourg. She moved to Finland on 2018 to join Prof. Hollanti’s group as a postdoc at the Mathematics Department at Aalto University. 2019 she joined Prof. Jung’s group and moved to the Computer Science Department. She is also Finland’s coordinator of European Women in Mathematics.
Dr. Carafi´s research interests include algebraic number theory, isogeny-based cryptography, wireless communications security and machine learning applications. She likes combining different unrelated areas to come up with new ideas.
-Number theory can be applied to security in wireless communications and to cryptography. Machine learning provides a new and exciting source to approach these areas from a different point of view, she says.
Achievements in 2020
-In 2020, we started to obtain the first results using deep learning to improve wireless communications in specific cases. This was a first step to a more general project that we’re currently working on, tells Carafi. These first results can be found in a paper accepted in the IEEE ICASSP 2021 conference.
-In the same area, but with a more classical approach, we got an accepted paper to appear in IEEE Transactions on Information Theory, Well-Rounded Lattices. Towards Optimal Coset Codes for Gaussian and Fading Wiretap Channels. Besides that I finished a project on homomorphic encryption started at the Private AI Bootcamp offered by Microsoft Research in December 2019, which resulted in a publication: Gimme That Model!: A Trusted ML Model Trading Protocol, to appear in Protecting Privacy through Homomorphic Encryption by Springer. I also finished a paper on isogeny-based cryptography, Explicit connections between supersingular isogeny graphs and Bruhat-Tits trees. A paper from my PhD thesis was finally accepted, Images of Galois representations in local mod p Hecke algebras. A paper from my PhD thesis was finally accepted, Images of Galois representations in local mod p Hecke algebras, tells Carafi.

Antti Hyttinen
Google scholar: https://scholar.google.fi/citations?user=38R60jAAAAAJ&hl=en
Adj. Prof. Antti Hyttinen obtained his Master’s degree Information Technology in Tampere University (of Technology) in 2004 and his PhD in Computer Science in 2013 from the University of Helsinki. His doctoral studies were supervised by Adj. Prof. Patrik Hoyer. Dr. Hyttinen did research at California Institute of Technology during 2014. Dr. Hyttinen has also obtained the competitive personal post-doc funding from the Academy of Finland (2016). Currently, Dr. Hyttinen works as a university researcher in the Sums of Products research group, collaborating with several research groups at the department.
Hyttinen’s research focuses on causal inference and probabilistic graphical models. Dr. Hyttinen has developed theory and structure learning algorithms for several different types of probabilistic graphical models. Many of these are causal models that allow also for latent confounding and feedback. These methods build on many different kinds of techniques such as Branch and Bound, MIP, MaxSAT, ASP, and dynamic programming. In addition, Dr. Hyttinen has developed methods for causal effect identification, experiment selection and combining different types of data sources for the aforementioned tasks.
Dr. Hyttinen received the title of docent (adjunct professor) in February 2020. During 2020, Dr. Hyttinen published a good number of papers with different collaborators, several papers in competitive venues such as AAAI and NeurIPS. Dr Hyttinen co-authored the article receiving the best paper award at the Discovery Science conference. Dr. Hyttinen also participated in research and thesis supervision at Ph.D., M.Sc., and B.Sc. levels.
Sample of recent publications:
J. Viinikka, A. Hyttinen, J. Pensar, M. Koivisto: Towards Scalable Bayesian Learning of Causal DAGs. In Advances in Neural Information Processing Systems 33, NeurIPS 2020.
K. Rantanen, A. Hyttinen, M. Järvisalo: Discovering Causal Graphs with Cycles and Latent Confounders: An Exact Branch-and- Bound Approach, International Journal of Approximate Reasoning, Volume 117, p. 29–49, 2020.
Riku Laine, Antti Hyttinen and Michael Mathioudakis:
Evaluating Decision Makers over Selectively Labeled Data: A Causal Modelling Approach In Proceedings of the 23rd International Conference on Discovery Science, 2020.

Naser Hossein Motlagh
Dr. Naser Hossein Motlagh received the B.Sc. degree in Information Technology and the M.Sc. degree in Telecommunications Engineering from the University of Vaasa, Finland, in 2012. Dr. Motlagh received his D.Sc. degree in networking technology from the Department of Communications and Networking, School of Electrical Engineering, Aalto University, Finland, in 2018. His doctoral research was focused on exploring solutions for challenges associated with Internet of Thing service delivery using Unmanned Aeraila vehicles (UAVs). In 2018, after receiving the D.Sc. degree, he continued as Postdoctoral researcher at the Department of Industrial Engineering and Management at Aalto University. From January 2019 to December 2020, he was a Postdoctoral fellow within Helsinki Institute for Information Technology (HIIT) – Helsinki Center for Data Science (HiDATA) program. From January 2019, he has been a member of MegaSense program and since January 2021, he is a Postdoctoral fellow within Nokia Center for Advanced Research (NCAR) at the University of Helsinki. For more information about Dr. Motlagh, please see his profile page at the University of Helsinki: https://researchportal.helsinki.fi/en/persons/naser-hossein-motlagh
Dr. Motlagh’s research is focused on large-scale deployment of sensor networks and providing Internet of Things services through data analytics at the edge of wireless communication networks. In general, his research interests include the Internet of Things (IoT), wireless sensor networks (WSN), environmental sensing, and unmanned aerial and underwater vehicles (UAV & AUVs); with focus on Networks, Platforms, Sensing, Applications and Services. Dr. Motlagh’s research aims to contribute smart and sustainable solutions and assist solving current and future problems of different environments such as by buildings energy optimization, marine pollution monitoring, and air pollution hotspot detection and monitoring in urban areas. Part of his research includes finding intelligent solutions in smart buildings. His research aims at exploring energy efficient power consumptions for buildings using the environmental factors such as light, temperature, and humidity, while offering thermal and visual comforts for the users. He also seeks novel IoT-based methodologies in smart environments for having healthy work and living spaces. He is carrying out research on large-scale autonomous marine pollution monitoring using coordinated groups of autonomous underwater vehicles (AUV)s to monitor the extent and characteristics of marine pollutants. In addition, with the use of low-cost air quality sensors and emerging communication technologies such as 5G and beyond networks, he explores solutions for challenges related to enabling massive scale deployments of air quality sensors in urban areas and seeking solutions for healthier living environemnts.
Dr. Motlagh has published eight journal articles and six conferences papers during his postdoctoral fellowship with Helsinki Institute for Information Technology (HIIT). For example, by publishing the MegaSense vision in the top venues such as IEEE Communications Magazine, IEEE Internet Computing Magazine, IEEE Internet of Things Magazine and IEEE Sensors Journal. His contribution to a conference paper was also among the top 5 papers in Proceedings of the 15th IEEE Conference on Industrial Electronics and Applications. He was also awarded a fellowship at Nokia Center for Advanced Research (NCAR).
Sample of recent publications:
Motlagh, N.H., Lagerspetz, E., Nurmi, P., Li, X., Varjonen, S., Mineraud, J., Siekkinen, M., Rebeiro-Hargrave, A., Hussein, T., Petaja, T. and Kulmala, M., 2020. Toward massive scale air quality monitoring. IEEE Communications Magazine, 58(2), pp.54-59.
Su, X., Liu, X., Motlagh, N.H., Cao, J., Su, P., Pellikka, P., Liu, Y., Petaja, T., Kulmala, M., Hui, P. and Tarkoma, S., 2021. Intelligent and Scalable Air Quality Monitoring with 5G Edge. IEEE Internet Computing.
Zaidan, M.A., Motlagh, N.H., Fung, P.L., Lu, D., Timonen, H., Kuula, J., Niemi, J.V., Tarkoma, S., Petäjä, T., Kulmala, M. and Hussein, T., 2020. Intelligent calibration and virtual sensing for integrated low-cost air quality sensors. IEEE Sensors Journal, 20(22), pp.13638-13652.
Motlagh, N.H., Zaidan, M.A., Lagerspetz, E., Varjonen, S., Toivonen, J., Mineraud, J., Rebeiro-Hargrave, A., Siekkinen, M., Hussein, T., Nurmi, P. and Tarkoma, S., 2019, July. Indoor air quality monitoring using infrastructure-based motion detectors. In 2019 IEEE 17th International Conference on Industrial Informatics (INDIN) (Vol. 1, pp. 902-907). IEEE.
Rebeiro-Hargrave, A., Motlagh, N.H., Varjonen, S., Lagerspetz, E., Nurmi, P. and Tarkoma, S., 2020, November. MegaSense: Cyber-physical system for real-time urban air quality monitoring. In 2020 15th IEEE Conference on Industrial Electronics and Applications (ICIEA) (pp. 1-6). IEEE.
Flores, H., Motlagh, N.H., Zuniga, A., Liyanage, M., Passananti, M., Tarkoma, S., Youssef, M. and Nurmi, P., 2021. Toward large-scale autonomous marine pollution monitoring. IEEE Internet of Things Magazine, 4(1), pp.40-45.

Razane Tajeddine
Dr. Razane Tajeddine received her B.Sc. in Electrical Engineering from Notre Dame University-Louaize, Faculty of Engineering, Lebanon, in 2012, and her M.Sc. in Electrical and Computer Engineering from the American University of Beirut, Lebanon, in 2014. She received her doctorate degree from the department of Mathematics and Systems Analysis at Aalto University in 2019. From 2014 to 2017, she was a PhD student at the Electrical and Computer Engineering Department at the Illinois Institute of Technology. In 2016, she came for a research visit to Aalto University to work in collaboration with the ANTA group. She was invited to the Algebraic Coding Theory for Networks, Storage, and Security, Dagstuhl in December 2018 and to the Oberwolfach Workshop on Contemporary Coding Theory, Leibniz in March 2019. Currently, Tajeddine is working as an HIIT Postdoctoral researcher at the University of Helsinki.
Research interests
Tajeddine’s research focuses on developing effective privacy preserving methods for machine learning, mainly where data is partitioned between multiple parties. Her doctoral research focused on private information retrieval (PIR), where schemes were developed to allow users to retrieve a file from a distributed storage system without revealing what file is being retrieved.
Achievements
During the year 2020, Tajeddine published a preprint for the work on private data sharing on vertically partitioned data, and presented the work as a poster in the AI day 2020.
Tajeddine, R., Jälkö, J., Kaski, S., & Honkela, A. (2020). Privacy-preserving Data Sharing on Vertically Partitioned Data. arXiv preprint arXiv:2010.09293.

Ashwin Rao
Ashwin Rao obtained his master’s degree in Information Technology in 2009 from the Indian Institute of Technology Delhi, and his PhD in Informatique (Computer Science) in 2013 (thesis defended in 2013 at Inria and certificate awarded in 2015) from University of Nice Sophia Antipolis, France. His doctoral studies were supervised by Professor Walid Dabbous and Professor Arnaud Legout. After graduating he arrived in Finland in July 2014 and has been a post-doctoral researcher since. He received the title of Docent from the University of Helsinki in September 2020.
He is actively teaching at the university, for example, co-supervising Bachelor’s, Master’s, and PhD theses.
As of April 2021, he has co-supervised 1 PhD student, 10 Masters Students, and co-hosted research visits of 2 PhD students. More information about his research activities can be found on his webpage.
His research interests are in the area of distributed systems and networking, and his projects have touched mobile networks, video streaming, peer-to-peer communication, wireless networks, privacy and security issues, and recently operating system architectures. His research works are heavily systems-oriented, and they involve building systems for conducting large-scale measurement studies, analyzing the results, and addressing the issues identified.
His current research is along the following two interrelated threads.
1. Parallelism for high performance computing. This work focuses on building an operating system which can be leveraged for I/O-intensive parallel tasks. It is motivated by the fact that the OS kernel needs to be bypassed for I/O operations because CPU speeds have stagnated while I/O speeds are increasing.
2. Multi-connectivity. Our devices use static rules for selecting the communication interface to use, and this work is focused on addressing this shortcoming and enabling the devices to coordinate with the network controllers managing the network functions in the network.
Achievements in 2020
He received the title of Docent in September 2020. He helped organize two webinars on Edge Intelligence which had an audience of more than 50 people. He co-authored a research proposal, IDEA-MILL, that received a 3-year funding from the Academy of Finland with Prof. Sasu Tarkoma as PI. In 2020, he also taught two masters level courses: Networked Systems and Services (5 cr), and Distributed Systems (5 cr), he was an instructor for 5 Bachelors Thesis, a co-supervisor for 2 Master’s Thesis, and 1 PhD student successfully defended his PhD thesis under his co-supervision.
Key Peer Reviewed Publications in 2020/21:
• Pozza, M., Rao, A., Lugones, D. F., & Tarkoma, S. (2021). FlexState: Flexible State Management of Network Functions. IEEE Access, 9, 46837-46850 (JUFO-2).
• Pozza, M., Nicholson, P. K., Lugones, D. F., Rao, A., Flinck, H., & Tarkoma, S. (2020). On Reconfiguring 5G Network Slices. IEEE Journal on Selected Areas in Communications, 38(7), 1542-1554 (JUFO-3).
• Hoque, M. A., Rao, A., & Tarkoma, S. (2021). Network and Application Performance Measurement Challenges on Android Devices. ACM SIGMETRICS Performance Evaluation Review, 48(3), 6-11.
• Hätönen, S., ul Huque, M. T. I., Rao, A., Jourjon, G., Gramoli, V., & Tarkoma, S. (2020). An SDN Perspective on Multi-Connectivity and Seamless Flow Migration. IEEE Networking Letters, 2(1), 19-22.
Key Technical Reports published in 2020/21
• Peltonen, E., et al. (2020). 6G White Paper on Edge Intelligence. (2020). 6G White Paper on Edge Intelligence.
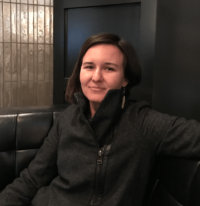
Joanna Slawinska
Google scholar: https://scholar.google.com/citations?user=aZF1u50AAAAJ
Dr Joanna Slawinska is a senior researcher at the Department of Computer Science, University of Helsinki, since December 2020. She has a masters degree in physics with a focus on asteroseismology (graduated in 2005) and also PhD in computational geophysics (graduated in 2011) from University of Warsaw, and postdoctoral research training in applied mathematics.
Joanna Slawinska has extensive expertise on data-driven methods for dynamical systems, and their applications to various fields of physics, such as extraction and predictions of spatiotemporal patterns in turbulent flows. Current and future interests include AI approaches to complex systems, in particular probabilistic and Bayesian methods that are relevant to understand further and improve predictability of high-dimensional time-resolved datasets, such as temporal networks, causality, or game theory.
Joanna Slawinska has joined University of Helsinki in December 2020. Since then, she has started collaborating on a number of projects, including with group of prof. Maarit Kapyla from Aalto University. She is interested in developing research agenda and collaborations with a group of prof. Teemu Roos on a number of topics, with special focus on Bayesian and probabilistic methods such as temporal networks, causality and game theory.
Prior to that, Joanna Slawinska has worked on a number of projects during 2020, including schemes for probabilistic predictions with novel scheme for quantum mechanics data assimilation scheme for dynamical systems. Draft is currently under preparation for submission. Another two projects that got completed and published in 2020 concern nonparameteric prediction of tropical dynamics and also novel decomposition and spatiotemporal pattern extraction in computational neuroscience.
Relevant publications
• N. Marrouch, J. Slawinska, D. Giannakis, and H. L. Read. Data-driven Koopman operator approach for computational neuroscience. Ann. Math. Artif. Intel., 88:1155–1173, 2019. doi:10.1007/s10472-019- 09666-2.
• X. Wang, J. Slawinska, and D. Giannakis. Extended-range statistical ENSO prediction through operator-theoretic techniques for nonlinear dynamics. Sci. Rep., 10:2636, 2020. doi:10.1038/s41598- 020-59128-7.
D. Giannakis, A. Ourmazd, J. Schumacher, and J. Slawinska. Quantum compiler for classical dynamical systems, 2020. URL https://arxiv.org/abs/2012.06097.

Nitin Williams
Google scholar: https://scholar.google.com/citations?user=mOWxh9MAAAAJ&hl=en
Dr. Nitin Williams completed his Ph.D. from University of Reading, UK, in 2011, on developing novel signal processing methods to analyse human neuroscience data. He continued this line of research during his first post-doctoral period under Prof. Richard Henson at MRC Cognition & Brain Sciences Unit, University of Cambridge, UK. This period resulted in several important publications, for e.g. in Nature Communications, Brain, Human Brain Mapping, from his involvement on the large Cam-CAN project. In 2015, he moved to Finland for his second post-doctoral period, under Prof. Matias Palva in Neuroscience Center, University of Helsinki, Finland. He was involved in an important project funded by the EU Flagship Human Brain Project, on investigating the functional connectome of the human brain, i.e. the set of connections between each pair of distinct brain regions. Towards this end, he has developed novel methods to analyse the connectome, and used these methods to uncover its modular organization.
Current research
Nitin is currently funded by HIIT, working with Prof. Samuel Kaski in Department of Computer Science, Aalto University, Finland. His project is on using Approximate Bayesian Computation (ABC) methods to estimate parameters of biologically plausible models of human neuroscience data. Current methods to analyse human neuroscience use abstract statistical models, hence do not furnish information about biological mechanisms producing these data. During his exciting project, Nitin will 1.) Build biologically plausible models of human neuroscience data, and 2.) Fit these models to experimental neuroscience data using ABC methods. Models and methods from the project will advance Cognitive Neuroscience, by providing researchers with tools to gain insight into mechanisms underpinning their data.
Achievements in 2021
In 2020, Nitin completed a 3-month Academy of Finland funded mobility period to Institute of Mathematical Science (IMSc), Chennai. During this period, he gained experience of building biologically plausible models of brain activity with Prof. Sitabhra Sinha, who is a renowned researcher in this field. Together with the HIIT funding, the work done in this period has resulted in a model, which produces brain networks closely corresponding to those observed in experimentally recorded data. Nitin will submit this work to NeuroImage in May 2021, and submit model implementations from the project, to open-source Brain Dynamics Toolbox (https://bdtoolbox.org/).